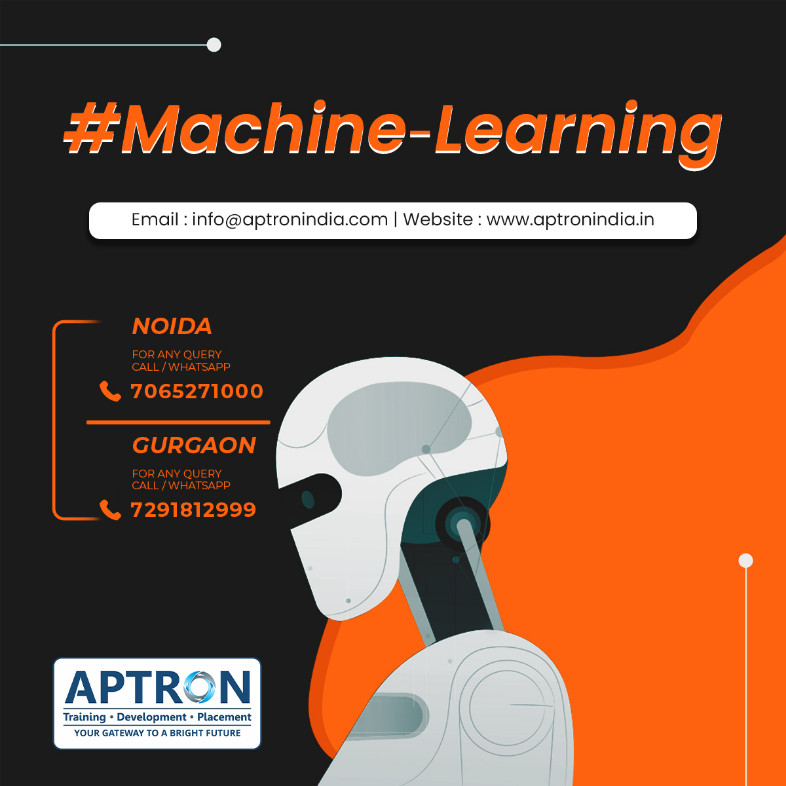
The idea that machines can replicate or even exceed human thinking has served as the inspiration for advanced computing frameworks – and is now seeing vast investment by countless companies. At the center of this concept are artificial intelligence (AI) and machine learning (ML).
These terms are often used synonymously and interchangeably. In reality, AI and ML represent two different things—though they are related. In essence:
Artificial intelligence can be defined as a computing system’s ability to imitate or mimic human thinking and behavior.
Machine learning, a subset of AI, refers to a system that learns without being explicitly programmed or directly managed by humans.
Today, both AI and ML play a prominent role in virtually every industry and business. They drive business systems and consumer devices. Natural language processing, machine vision, robotics, predictive analytics and many other digital frameworks rely on one or both of these technologies to operate effectively.
Brief History of AI and ML
The idea of building machines that think like humans has long fascinated society. During the 1940s and 1950s, researchers and scientists, including Alan Turing, began to explore the idea of creating an “artificial brain.” In 1956, a group of researchers at Dartmouth College began to explore the idea more thoroughly. At a workshop held at the university, the term “artificial intelligence” was born.
Over the following few decades, the field advanced. In 1964, Joseph Weizenbaum in the MIT Artificial Intelligence Laboratory invented a program called ELIZA. It demonstrate the viability of natural language and conversation on a machine. ELIZA relied on a basic pattern matching algorithm to simulate a real-world conversation.
During the 1980s, as more powerful computers appeared, AI research began to accelerate. In 1982, John Hopfield showed that a neural network could process information in far more advanced ways. Various forms of AI began to take shape, and the first artificial neural network (ANN) appeared in 1980.
During the last two decades, the field has advanced remarkably, thanks to enormous gains in computing power and software. AI and now ML is now widely used in a wide array of enterprise deployments. These technologies are used in natural language systems like Siri and Alexa, autonomous vehicles and robotics, automated decision-making systems in computer games, recommendation engines like Netflix, and extended reality (XR) tools, such as virtual reality (VR) and augmented reality (AR).
Machine learning in particular has flourished. It is increasingly used by government entities, businesses and others to identify complex and often elusive patterns involving statistics and other forms of structured and unstructured data. This includes areas as diverse as epidemiology and healthcare, financial modeling and predictive analytics, cybersecurity, chatbots and other tools used for customer sales and support. In fact, many vendors offer ML as part of cloud and analytics applications.
What Is the Impact of Artificial Intelligence?
A machine’s ability to emulate human thinking and behavior profoundly changes the relationship between these two entities. AI unleashes automation at scale and enables an array of more advanced digital technologies and tools, including VR, AR, digital twins, image and facial recognition, connected devices and systems, robotics, personal assistants and a variety of highly interactive systems.
This includes self-driving cars that navigate real-world conditions, smart assistants that answer questions and switch lights on and off, automated financial investing systems, and airport cameras and facial recognition. The latter includes biometric boarding passes airlines use at departure gates and the Global Entry system that requires only a face scan to pass through security checkpoints.
Indeed, businesses are putting AI to work in new and innovative ways. For example, dynamic pricing models used by the travel industry gauge supply and demand in real-time and adjusts pricing for flights and hotels to reflect changing conditions.
AI technology is used to better understand supply change dynamics and adapt sourcing models and forecasts. In warehouses, machine vision technology (which is supported by AI) can spot things like missing pallets and manufacturing defects that are too small for the human eye to detect. Meanwhile, chatbots analyze customer input and provide contextually relevant answers on a live basis.
Not surprisingly, these capabilities are advancing rapidly—especially as connected systems are added to the mix. Smart buildings, smart traffic grids and even smart cities are taking shape. As data streams in, AI systems determine the next optimal step or adjustment.
Similarly, digital twins are increasingly used by airlines, energy firms, manufacturers and others to simulate actual systems and equipment and explore various options virtually. These advanced simulators predict maintenance and failures but also provide insight into less expensive and more sophisticated ways to approach business.
What Is the Impact of Machine Learning?
Machine learning has also advanced remarkably in recent years. Using statistical algorithms, machine learning unlocks insights that have traditionally been associated with data mining and human analysis.
Using sample data, referred to as training data, it identifies patterns and applies them to an algorithm, which may change over time. Deep learning, a type of machine learning, uses artificial neural networks to simulate the way the human brain works.
These are the primary ways to use ML:
Supervised learning, which requires a person to identity the desirable signals and outputs.
Unsupervised learning, which allows the system to operate independent of humans and find valuable output.
Semi-supervised learning and reinforcement learning, which involves a computer program that interacts with a dynamic environment to achieve identified goals and outcomes. An example of the latter is a computer chess game. In some cases, data scientists use a hybrid approach that combines elements of more than one of these methods.
A Variety of Algorithms
Several types of machine learning algorithms play a key role:
Neural Networks: Neural networks simulate the way the human brain thinks. They’re ideal for recognizing patterns and they are widely used for natural language processing, image recognition and speech recognition.
Linear Regression: The technique is valuable for predicting numerical values, such as predicting prices for flights or real estate.
Logistic regression: This method typically uses a binary classification model (such as “yes/no”) to tag or categorize something. A common use for this technology is identifying spam in email and blacklisting unwanted code or malware.
Clustering: This ML tool uses unsupervised learning to spot patterns that humans may overlook. An example of clustering is how a supplier performs for the same product at different facilities. This approach might be used in healthcare, for instance, to understand how different lifestyle conditions impact health and longevity.
Decision Tree: The approach predicts numerical values but also performs classification functions. It delivers a clear way to audit results, unlike other forms of ML. This method also works with Random Forests, which combine Decision Trees.
Regardless of the exact method, ML is increasingly used by companies to better understand data and make decisions. This, in turn, feeds more sophisticated AI and automation. For example, sentiment analysis plugs in historical data about sales, social media data and even weather conditions to adapt manufacturing, marketing, pricing and sales tactics dynamically. Other ML applications deliver recommendation engines, fraud detection and image classification used for medical diagnostics.
One of the strengths of machine learning is that it can adapt dynamically as conditions and data change, or an organization adds more data. As a result, it’s possible to build an ML model and then adapt it on the fly. For example, a marketer might develop an algorithm based on a customer’s behavior and interests and then adapt messages and content as the customer changes his or her behavior, interests or purchasing patterns.
How are AI and ML Evolving in the Enterprise?
As mentioned, most software vendors—across a wide spectrum of enterprise applications—offer AI and ML within their products. These systems make it increasingly simple to put powerful tools to work without extensive knowledge of data science.
Yet, there are some caveats. For customers, in order to get the most out of AI and ML systems, an understanding of AI and some expertise is often necessary. It’s also vital to avoid vendor hype when selecting products. AI and ML can’t fix underlying business problems—and in some instance, they can produce new challenges, concerns and problems.
What are the Ethical and Legal Concerns?
AI and ML are at the center of a growing controversy—and they should be used wisely—and carefully. They have been associated with hiring and insurance bias, racial discrimination and a variety of other problems, including misuse of data, inappropriate surveillance and things like deep fakes and false news and information.
There’s growing evidence that facial recognition systems are considerably less accurate when identifying people of color—and they can lead to racial profiling. Moreover, there are growing concerns about governments and other entities using facial recognition for mass surveillance. So far, there’s very little regulation of AI practices. Yet Ethical AI is emerging as a key consideration.
What is the Future of ML and AI?
AI technologies are advancing rapidly, and they will play an increasingly prominent role in the enterprise—and our lives. AI and ML tools can trim costs, improve productivity, facilitate automation and fuel innovation and business transformation in remarkable ways.
As the digital transformation advances, various forms of AI will serve as the sun around which various digital technologies orbit. AI will spawn far more advanced natural speech systems, machine vision tools, autonomous technologies, and much more.
Also see: Top Digital Transformation Companies
What is machine learning, and why is it crucial for your digital transformation strategy?
Machine learning (ML) is a type of artificial intelligence (AI) that can help businesses understand, organize, and act upon company and customer data with greater speed and accuracy, and with significantly fewer costs. In doing so, ML helps empower business units to be proactive, innovative, productive, and customer-centric: hallmarks of a successful digital transformation.
In this article, we provide a definition of machine learning, and explain why it’s important for businesses and their digital transformation strategies. We’ll share examples of some benefits of machine learning DevOps, as well as ML for customer service, operations, and sales and marketing strategies.
What is machine learning?
With traditional artificial intelligence, specific, usually rule-based outcomes are written directly into a program.
For example, rudimentary AI-based chatbots can have simple conversations with a human based on a set of rules that has been provided to it. Somewhere in the software, a human has supplied the rule that when the chatbot sees the word accounting, it should provide a list of predetermined answers relating to accounting. The signal (accounting), rule, and outcomes were provided by a human. They are hardwired in.
Machine learning, on the other hand, enables a computer program to develop its own set of rules from data that has been provided to it.
The developers of a more sophisticated ML chatbot might provide it with millions of real-world conversations between clients and customer service representatives (what’s known as training data). The chatbot would then search for patterns in the conversations and develop its own set of rules based on them. There’s no need for a human developer to provide the chatbot with rules or direction.
The more conversations the chatbot has, the more sophisticated and accurate its responses. An important caveat is that ML is only as accurate and unbiased as the information it’s given, which is why data quality is important when training ML software.
Why is machine learning essential for digital transformation?
Digital transformation can be defined as the re-conceptualization and restructuring of business practices, operations, and culture through the reasoned integration and adoption of digital tools, typically with a highly customer-centric focus.
Machine learning excels at rapidly organizing, analyzing, and making intelligent decisions and recommendations based on large quantities of data. It empowers employees and business leaders to make full use of company data, and to do so much more quickly, easily and comfortably than ever before. This frees team leaders and members from mundane, repetitive or complex and time-consuming tasks, enabling them to focus on meeting business objectives and provide outstanding customer experience.
In addition, there are examples where machine learning now outperforms humans. When it comes to understanding evolving customer needs, for instance, determining the next best action in a sales experience, or ensuring resources are allocated in response to business needs, machine learning is often better able to manage these tasks.
Digital transformation is about empowering human workers to be highly creative, fully engaged and productive, and laser-focused on the customer experience. Machine learning tools can help make this possible by providing employees with the right information at the right time, in formats that make sense for their role, and in a way that ties together business units.
How businesses are benefiting from machine learning
Here are a few examples of how to use machine learning successfully across business units.
Understanding customers. In recent years, the quantity of client data available to businesses has increased significantly. As a result, data has become more difficult to analyze and understand without the help of machines. A variety of ML-based tools and platforms now exist that help businesses gain a more profound understanding of their customers. This has led to better customer experiences, especially for teams in marketing, customer services, and sales.
IT, DevOps and incident management. Most businesses use a wide range of technologies and digital tools for day-to-day operations. IT and DevOps teams keep everything running smoothly. Yet, as digital tools become common and their interactions more complex, the number of technical incidents increases. Machine learning can be used to reduce noise, gain a better understanding of relationships between incidents, and reduce time to resolution.
Supply chain, production, and logistics. This is one area where the adoption of machine learning has led to significant cost reductions for many businesses. BMW, for example, uses ML-based algorithms to move 31 million individual parts around its factories in response to supply and demand. Freed of this logistical nightmare, BMW’s employees can focus on customer relations, design improvements, and more.
Human resources and people management. Many of the most popular HR and people management platforms leverage machine learning to streamline this complex task. Importantly, ML enables smaller, leaner HR teams to manage a larger number of employees. ML-based chatbots can respond to most typical employee queries, which saves time and reduces the chance of human error.
Understanding market trends. Given the right data, machines can be quite accurate at predicting human behavior and spotting the emergence of new market trends. In fact, there are a variety of ways machines are now outperforming humans and traditional AI in forecasting, be it for hurricanes, earthquakes, or business markets.
A successful digital transformation strategy should focus on empowering employees and business leaders. It should equip them with digital tools that simplify business processes, encourage innovation and creativity, and provide a fuller understanding of the customer experience.
Machine learning, by virtue of its ability to rapidly and intelligently analyze company and customer data, can do just this. Businesses are already using machine learning to augment a variety of business units and successfully undergo digital transformations.
TechRadar created this content as part of a paid partnership with PagerDuty. The contents of this article are entirely independent and solely reflect the editorial opinion of TechRadar.
Artificial Intelligence and Machine Learning: Enhancing Human Effort with Intelligent Systems
Only when the challenges of data accessibility and expensive computing power were mitigated did the AI field experience exponential growth. There are now more than a dozen types of AI being advanced. This feature originally appeared in InTech magazine's August issue, a special edition from ISA's Smart Manufacturing and IIoT Division.
Artificial Intelligence and Machine Learning: Enhancing Human Effort with Intelligent Systems
Artificial intelligence has come a long way since scientists first wondered if machines could think.
In the 20th century, the world became familiar with artificial intelligence (AI) as sci-fi robots who could think and act like humans. By the 1950s, British scientist and philosopher Alan Turing posed the question “Can machines think?” in his seminal work on computing machinery and intelligence, where he discussed creating machines that can think and make decisions the same way humans do (Reference 1). Although Turing’s ideas set the stage for future AI research, his ideas were ridiculed at the time. It took several decades and an immense amount of work from mathematicians and scientists to develop the field of artificial intelligence, which is formally defined as “the understanding that machines can interpret, mine, and learn from external data in a way that imitates human cognitive practices”
Even though scientists were becoming more accustomed to the idea of AI, data accessibility and expensive computing power hindered its growth. Only when these challenges were mitigated after several “AI winters” (with limited advances in the field) did the AI field experience exponential growth.
Due to the accelerated popularity of AI in the 2010s, venture capital funding flooded into a large number of startups focused on machine learning (ML). This technology centers on continuously learning algorithms that make decisions or identify patterns. For example, the YouTube algorithm may recommend less relevant videos at first, but over time it learns to recommend better targeted videos based on the user’s previously watched videos.
The three main types of ML are supervised, unsupervised, and reinforcement learning. Supervised learning refers to an algorithm finding the relationship between a set of input variables and known labeled output variable(s), so it can make predictions about new input data. Unsupervised learning refers to the task of intelligently identifying patterns and categories from unlabeled data and organizing it in a way that makes it easier to discover insights. Lastly, reinforcement learning refers to intelligent agents that take actions in a defined environment based on a certain set of reward functions.
Deep learning, a subset of ML, had numerous ground-breaking advances throughout the 2010s. Similar to the connections between the nervous system cells in the brain, neural networks consist of several thousand to a million hidden nodes and connections. Each node acts as a mathematical function, which, when combined, can solve extremely complex problems like image classification, translation, and text generation.
Impact of artificial intelligence
Human lifestyle and productivity have drastically improved with the advances in artificial intelligence. Health care, for example, has seen immense AI adoption with robotic surgeries, vaccine development, genome sequencing, etc. (Reference 5). So far, the adoption in manufacturing and agriculture has been slow, but these industries have immense untapped AI possibilities (Reference 6). According to a recent article published by Deloitte, the manufacturing industry has high hopes for AI because the annual data generated in this industry is thought to be around 1,800 petabytes.
This proliferation in data, if properly managed, essentially acts as a “fuel” that drives advanced analytical solutions that can be used for the following (Reference 8):
becoming more agile and disruptive by learning trends about customers and the industry ahead of competitors
saving costs through process automation
improving efficiency by identifying processes’ bottlenecks
enhancing customer experience by analyzing human behavior
making informed business decisions, such as targeted advertising and communication
Ultimately, AI and advanced analytics can augment humans to help mitigate repetitive and sometimes even dangerous tasks while increasing focus on endeavors that drive high value. AI is not a far-fetched concept; it is already here, and it is having a substantial impact in a wide range of industries. Finance, national security, health care, criminal justice, transportation, and smart cities are examples of this.
AI adoption has been steadily increasing. Companies are reporting 56 percent adoption in 2021, an uptick of 6 percent compared to 2020 (Reference 10). With the technology becoming more mainstream, the trends of achieving solutions that emphasize “explainability,” accessibility, data quality, and privacy are amplified.
“Explainability” drives trust: To keep up with the continuous demand of more accurate AI models, hard-to-explain (black-box) models are used. Not being able to explain these models makes it difficult to achieve user trust and to pinpoint problems (bias, parameters, etc.), which can result in unreliable models that are difficult to scale. Due to these concerns, the industry is adopting more explainable artificial intelligence (XAI).
According to IBM, XAI is a set of processes and methods that allows human users to comprehend and trust the ML algorithm’s outputs (Reference 11). Additionally, explainability can increase accountability and governance.
Increasing AI accessibility: The “productization” of cloud computing for ML has taken the large compute resources and models, once reserved only for big tech companies, and put them in the hands of individual consumers and smaller organizations. This drastic shift in accessibility has fueled further innovation in the field. Now, consumers and enterprises of all sizes can reap the benefits of:
pretrained models (GPT3, YOLO, CoCa [finetuned])
building models that are no-code/low-code solutions (Azure’s ML Studio)
serverless architecture (hosting company manages the server upkeep)
instantly spinning up more memory or compute power when needed
improved elasticity and scalability.
Data mindset shift: Historically, model-centric ML development, i.e., “keeping the data fixed and iterating over the model and its parameters to improve performances” (Reference 12), has been the typical approach. Unfortunately, the performance of a model is only as good as the data used to train it. Although there is no scarcity of data, high-performing models require accurate, properly labeled, and representative datasets. This concept has shifted the mindset from model-centric development toward data-centric development—“when you systematically change or enhance your datasets to improve the performance of the model” (Reference 12).
An example of how to improve data quality is to create descriptive labeling guidelines to mitigate recall bias when using data labeling companies like AWS’ Mechanical Turk. Additionally, responsible AI frameworks should be in place to ensure data governance, security and privacy, fairness, and inclusiveness.
Data privacy through federated learning: The importance of data privacy has not only forged the path to new laws (e.g., GDPR and CCPA), but also new technologies. Federated learning enables ML models to be trained using decentralized datasets without exchanging the training data. Personal data remains in local sites, reducing the possibility of personal data breaches.
Additionally, the raw data does not need to be transferred, which helps make predictions in real time. For example “Google uses federated learning to improve on-device machine learning models like ‘Hey Google’ in Google Assistant, which allows users to issue voice commands”.
AI in smart factories
Maintenance, demand forecasting, and quality control are processes that can be optimized through the use of artificial intelligence. To achieve these use cases, data is ingested from smart interconnected devices and/or systems such as SCADA, MES, ERP, QMS, and CMMS. This data is brought into machine learning algorithms on the cloud or on the edge to deliver actionable insights. According to IoT Analytics (Reference 14), the top AI applications are:
predictive maintenance (22.2%)
quality inspection and assurance (19.7%)
manufacturing process optimization (13%)
supply chain optimization (11.5%)
AI-driven cybersecurity and privacy (6.6%)
automated physical security (6.5%)
resource optimization (4.8%)
autonomous resource exploration (3.8%)
automated data management (2.9%)
AI-driven research and development (2.1%)
smart assistant (1.6%)
other (5.2%).
Vision-based AI systems and robotics have helped develop automated inspection solutions for machines. These automated systems have not only been proven to save human lives but have radically reduced inspection times. There have been significant examples where AI has outperformed humans, and it is a safe bet to conclude that several AI applications enable humans to make informed and quick decisions.
Given the myriad additional AI applications in manufacturing, we cannot cover them all. But a good example to delve deeper into is predictive maintenance, because it has such a large effect on industry.
Generally, maintenance follows one of four approaches: reactive, or fix what is broken; planned, or scheduled maintenance activities; proactive, or defect elimination to improve performance; and predictive, which uses advanced analytics and sensing data to predict machine reliability.
Predictive maintenance can help flag anomalies, anticipate remaining useful life, and provide mitigations or maintenance. Compared to the simple corrective or condition-based nature of the first three maintenance approaches, predictive maintenance is preventive and takes into account more complex, dynamic patterns. It can also adapt its predictions over time as the environment changes. Once accurate failure models are built, companies can build mathematical models to reduce costs and choose the best maintenance schedules based on production timelines, team bandwidth, replacement piece availability and other factors.
Bombardier, an aircraft manufacturer, has adopted AI techniques to predict the demand of its aircraft parts based on input features (i.e., flight activity ) to optimize its inventory management.
This example and others show how advances in AI depend on advances associated with other Industry 4.0 technologies, including cloud and edge computing, advanced sensing and data gathering, and wired and wireless networking.
Computing Machinery and Intelligence
"A Complete History of Artificial Intelligence"
"Macht KI unsere Informationen intelligent?"
"Data is the New Currency. Don’t Let It Slip Through Your Fingers"
"No longer science fiction, AI and robotics are transforming healthcare"
"Technical and human factors are restricting AI adoption in manufacturing"
"Deloitte Survey on AI Adoption in Manufacturing"
"Utilizing AI and Big Data To Reduce Costs and Increase Profits In Departments Across An Organization"
"Advantages & Disadvantages of Big Data"
"The state of AI in 2021"
"Explainable AI"
"Data-Centric AI vs. Model-Centric AI? Which one will win?"
"What is Federated Learning (FL)? Techniques & Benefits in 2022"
IOT Analytics
"6 areas where artificial neural networks outperform humans"
"What is Predictive Maintenance?"
"How to Implement Machine Learning For Predictive Maintenance"
Bombardier Aftermarket Demand Forecast with Machine Learning
This feature originally appeared in InTech magazine's August issue, a special edition from ISA's Smart Manufacturing and IIoT Division.
Ines Mechkane is the AI Technical committee chair of ISA’s SMIIoT Division. She is also a senior technical consultant with IBM. She has a background in petroleum engineering and international experience in artificial intelligence, product management, and project management. Passionate about making a difference through AI, Mechkane takes pride in her ability to bridge the gap between the technical and business worlds.
Manav Mehra is a data scientist with the Intelligent Connected Operations team at IBM Canada focusing on researching and developing machine learning models. He has a master’s degree in mathematics and computer science from the University of Waterloo, Canada, where he worked on a novel AI-based time-series challenge to prevent people from drowning in swimming pools.
Adissa Laurent is AI delivery lead within LGS, an IBM company. Her team maintains AI solutions running in production. For many years, Laurent has been building AI solutions for the retail, transport, and banking industries. Her areas of expertise are time series prediction, computer vision, and MLOps.
Eric Ross is a senior technical product manager at ODAIA. After spending five years working internationally in the oil and gas industry, Ross completed his master of management in artificial intelligence. Ross then joined the life sciences industry to own the product development of a customer data platform infused with AI and BI.
Top 25 Machine Learning Interview Questions
What are the basic differences between Machine Learning and Deep Learning?
What is the difference between Bias and Variance?
What is the difference between supervised and unsupervised machine learning?
What are the three stages of model building in machine learning?
What are the applications of supervised machine learning?
What are the techniques of Unsupervised machine learning?
What are the different types of Machine Learning?
What is Deep Learning?
Comparison between Machine Learning and Big Data
Explain what is precision and Recall?
What is your favorite algorithm and also explain the algorithm briefly in a minute?
What is the difference between Type1 and Type2 errors?
Define what is Fourier Transform in a single sentence?
What is deep learning?
What is the F1 score?
How is the F1 score is used?
How can you ensure that you are not overfitting with a particular model?
How to handle or missing data in a dataset?
Do you have any relevant experience on Spark or any of the big data tools that are used for Machine Learning?
Pick an algorithm and write a Pseudocode for the same?
What is the difference between an array and a Linked list?
Define a hash table?
Mention any one of the data visualization tools that you are familiar with?
What is your opinion on our current data process?
Please let us know what was your last read book or learning paper on Machine Learning?
Which is the Best Machine Learning Training Institute in Noida
The most effective way is by joining APTRON Noida’s Online Machine Learning course or Machine Learning course in Noida. The Machine Learning course is designed in the most exclusive manner consisting of supervised and unsupervised algorithms efficiently. Upgrade your skills with Online Machine Learning course and make your job-ready whilst grasping the opportunity of most high-paying jobs via our placement cell.
A passion for working with cutting-edge technologies and these staggering stats alone are reasons enough to pursue a career in machine learning. Professionals all over the world are turning towards credible Online Machine Learning training or Machine Learning Training in Noida that impart practical knowledge. And, this ML course from Talentedge boasts of quality content and practical knowledge, all of which is enclosed in a structured learning approach wherein lectures are delivered LIVE via eminent faculty of top-rated institutions.